IDC Team Demonstrates the Value of AI in Generating Imaging Annotations
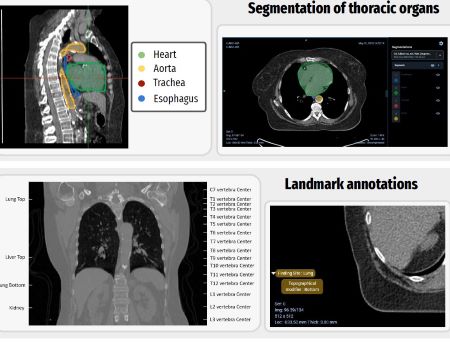
A team of researchers affiliated with the CRDC Imaging Data Commons (IDC) has demonstrated the value of AI in generating annotations for imaging data sets that are now better situated to serve as reference data sets for users across the cancer research community.
Enrichment of lung cancer computed tomography collections with AI-derived annotations, Krishnaswamy et al., was published in early January 2024, in Nature’s open access journal Scientific Data. The research team thoroughly demonstrates the process of working with two datasets that are now annotated and available through the IDC: the Non-Small Cell Lung Cancer (NSCLC) Radiomics dataset, and a subset of the National Lung Screening Trial (NLST) dataset. The paper describes their methodology and results in detail, and includes links to tutorials for working with these datasets and the tools developed to create these annotations. First author Deepa Krishnaswamy, PhD, is an Instructor in Radiology at Brigham and Women’s Hospital and Harvard Medical School.
Until this work was completed, the NSCLC-Radiomics collection contained labeled tumors, but only partially labeled organs of interest. The NLST dataset, though widely used by many researchers, did not contain any image annotations.
As the team notes, lung cancer researchers can now more easily use these collections as reference datasets for comparative analysis. There are many downstream applications and benefits to working with these two collections that include annotations about anatomical region segmentations, radiomics extraction from those regions, the localization of bone and organ landmarks, as well as the labeling of regions. Among them:
- Segmentation of anatomic structures is a common preprocessing step in image analysis pipelines. The work done here, including the algorithms and workflows used, can substantially reduce effort and expense for researchers using these reference datasets.
- Segmentation of thoracic structures, as done through this project, is necessary for the identification of organs-at-risk for radiotherapy treatment planning.
- Segmentations generated from the multiple nnU-Net models in this study enable the evaluation of the generalizability of the provided algorithms on an external dataset to establish a baseline for evaluation of alternative similar algorithms.
- Shape radiomics features from these datasets can be used to detect potential outliers in segmentations in comparative datasets, as quantitative features to stratify patients within a comparative cohort, or to contribute to applications that utilize such features in more complex analyses.
- Annotations of landmarks and regions can assist in defining regions of interest to simplify the task of segmentation of relevant structures.
- Given slice-level annotations of the landmarks and anatomic regions applied to these datasets, it is now possible to define anatomy-based search filters.
The annotated images, as well as the algorithms and workflows used, are all available publicly through the IDC forum with instructions about where to find data. The related GitHub Repository demonstrates the new dashboards and visualization tools.
All links:
Read the paper.
Find the datasets.
Read the IDC forum announcement.
Find the GitHub repository for this work.